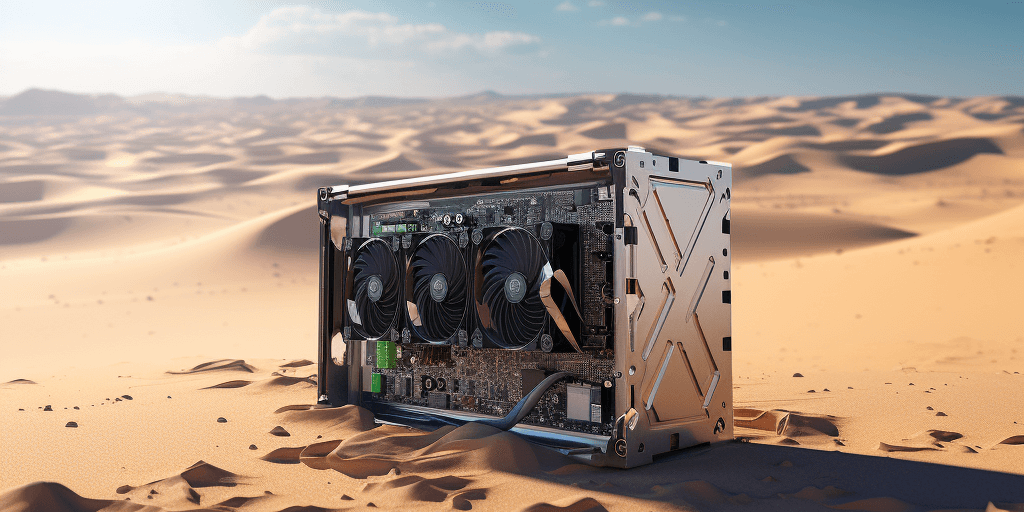
AI compute costs are eating up startups' runway just as fundraising is getting tougher. How can startups navigate the shortage to get the compute resources they need? How should they shop for compute providers across different clouds? And how can the industry keep up with demand without exacerbating climate change?
SignalFire brought together leaders in the compute space for a real-talk panel at our SF headquarters to lay out how startups can build with AI without breaking the bank. The top takeaways include:
- The AI compute shortage is being caused by the sudden spike in demand, complexity of building modern GPUs, and need for algorithmic solutions that enhance efficiency.
- Startups and other compute buyers should use a multi-cloud approach, testing which use cases perform best with which providers rather than trying to dodge egress costs using a one-cloud-fits-none approach.
- AI’s contributions to humanity make compute "energy well spent", and new approaches to data center cooling and software-based efficiency improvements will reduce power consumption and climate impact.
![]() |
Here are all the top insights on AI compute from our discussion with Nvidia Chief Platform Architect for data center products Robert Ober, Crusoe Energy Co-founder and CEO Chase Lochmiller, and MosaicML Co-founder and CEO Naveen Rao, hosted by SignalFire’s AI Lab lead Veronica Mercado. And if you’re building something special in AI and want access to help with compute, recruiting, data science, and marketing, SignalFire would be excited to talk to you!
Is there a compute shortage? Yes, but not because there aren't enough GPUs
It's just that they're all locked up in contracts. The hockey stick growth of ChatGPT and the AI sector in general has put massive stress on the whole semiconductor industry and supply chain. This has pushed companies that were ahead of the curve to reserve any available GPUs, which have doubled in price since 2020. So while you might be able to get pricing for a spot instance, vendors can't fulfill those allocations, and getting a cluster is next to impossible without inside connections.
Essentially, the software demand has massively outstripped our physical infrastructure for producing the hardware. Meanwhile, the complexity of chips, high-performance networking, and packaging has grown significantly, pushing prices and failure rates up, and yield down.
“You can't just press a button and build 10X more” —Nvidia's Robert Ober
Ober from Nvidia says the big cloud business leaders are asking to suddenly increase production by 10X, but he emphasizes that "this is real hardware. You can't just press a button and build 10X more . . . These are truly the most complex systems anyone's ever built." With demand and complexity growing quickly, scaling up compute manufacturing will take time. We'll need ways to improve maximum performance by optimizing what Mosaic calls "model flop utilization"—securely intermixing users so a given piece of hardware is running all the time.
![]() |
Algorithmic solutions may be our best hope of closing the gap between surging demand and lagging supply. Of course we'll continue to need advanced packaging innovations and better chips so we get more performance per watt and have more compute to apply. But algorithmic innovations have outpaced hardware improvements of late, and are our best chance for doing more with less given the hardware shortage.
How can customers optimize their AI compute spend? Experimenting across multi-cloud environments
Founders may be tempted to try to save money on training and deploying their models by configuring their own compute—building and running their own mini cluster garage network infrastructure. Instead, they're likely best off turning to a vendor that lives and breathes efficiency. But the desire to home-brew compute shows a failure of the cloud ecosystem, where big clouds should get such efficiencies of scale that they pass on that no one would want to do it themselves. Unfortunately, some large cloud providers bundle in managed services that startups don't actually need, and their cloud egress costs can be daunting.
Lochmiller of Crusoe says we're suffering from the "Hotel California cloud model"—you can check in your data anytime you want but you can never leave. Obnoxious egress fees can bully startups into sticking with one cloud provider. But the improved fit and flexibility of using different, smaller, specialized providers for different use cases is likely to outweigh those egress fees.
“Multi-cloud is of much greater value” —MosaicML's Naveen Rao
Due to differences in their internal network infrastructure, control planes, and instances, one cloud may be best for CNN inference, another for large language model inference, another for training a small model across a couple of nodes, and another for when you need 4000 GPUs. You might run training, workloads, and customer data in different places to get the best provider for each. And the compute itself is so expensive itself that the added fees are drops in the bucket. Startups can also use intermediaries that stream data across providers to seek out the highest efficiency. Rao from MosaicML said it found Amazon S3 was up to three times more expensive than the next competitor, so staying locked into a single name-brand cloud can be very costly.
![]() |
Rao breaks down the fallacy of egress and streaming costs, saying, "When you're training a large language learner like MPT-7B, it costs $200,000 in compute. About $800 of that is streaming. It's not that much, right? It's less than half a percent. The flexibility to have multi-cloud is of much greater value to you than the loss of streaming." So you and your startup should hunt around, check that you can actually get the instances you're promised, experiment to see what gets the best efficiency where, and repeat the process as your needs change and scale.
How do we minimize the climate impact of AI compute? Energy usage isn't bad, but it needs to be efficient
"If all this innovation accelerates the climate crisis, what's the point?" Lochmiller declares. The compute- and energy-intensive nature of AI has raised concerns about how its environmental impact could hasten climate change, which also threatens to sour public perception and invite onerous regulation.
Lochmiller says data centers represent 1 to 1.5% of global power consumption today. That was forecast to grow to 8% by 2030, but with the AI boom, he says it will probably hit 10% sooner than that. Our panelists agreed that demand for AI has been consistently growing about 10 times per year, yet we're only improving compute supply by three times.One thing that's not likely to save the planet right away is edge computing. Phones lack sufficient compute power to do learning at the edge, so tokenizing data to be sent to the cloud for processing will remain the norm.
“Energy well spent” —Nvidia's Robert Ober
Luckily, AI is latency-tolerant, since feeding tokens through a massive model already takes some time. This could lead to more data centers being built closer to where energy is cheap and abundant, since the added latency is less noticeable. New data center cooling systems that run cold water through copper pipes could also help by more efficiently transferring heat off of chips. Plus, this could reduce the use of energy-sucking HVAC units and high failure rate fans. Applying AI itself to designing more effective hardware could minimize electricity consumption.
![]() |
But overall, it's important to remember that “[powering AI is] energy well spent, because it allows us to do things that were impossible before," from genomics to autonomous vehicles, Ober insists. Lochmiller concludes that "people often conflate this idea that using energy is bad, that we should use less. It's actually the opposite. If you look at the correlation of the human development index to the amount of energy used, more advanced societies use more energy and it's going to continue to be the case. It's more a matter of how efficient we are."
SignalFire is AI-native venture firm that’s been building and refining its own models for a decade. Our Beacon AI data platform helps our investors spot amazing founders and helps our portfolio companies recruit the best talent. Along with our seed-to-Series B investment practice, we recently launched the SignalFire AI Lab to pair technology leaders and sector experts with corporates as data providers, design partners, and initial customers. We’d love to hear about what you’re building!
*Portfolio company founders listed above have not received any compensation for this feedback and may or may not have invested in a SignalFire fund. These founders may or may not serve as Affiliate Advisors, Retained Advisors, or consultants to provide their expertise on a formal or ad hoc basis. They are not employed by SignalFire and do not provide investment advisory services to clients on behalf of SignalFire. Please refer to our disclosures page for additional disclosures.
Related posts
