
What’s the best AI model for your use case? Will it work with no hallucinations? These questions are plaguing every enterprise trying to adopt AI.
This stems from a huge shift in how we build software. The first wave of machine learning tooling was about in-house experimentation and training, and for good reason. The workflow was complex and third-party infrastructure tooling was insufficient. Traditional ML work was focused on model architecture and training hyperparameters, all driving toward consistent performance with a company’s specific product.
![]() |
Foundation models changed everything
Using zero-shot learning for ML product development, we now have machine-learning-as-a-service. Enterprises can license and integrate an AI model without having to build a full-fledged MLOps and tooling stack. But which model should they choose? Now they need a rigorous testing solution to validate and compare the best model in the market for their use cases.
![]() |
Without a methodical way to verify performance, there are significant risks to adopting foundational models—LLM factualness, jailbreaking, privacy, hallucinations, and other characteristics. This is why the modern ML stack is test-driven. It’s all about gathering training data, fine-tuning models, and then checking that they consistently work as expected.
Kolena testing tools catalyze AI adoption
Kolena turns AI model comparison, testing, and validation into a science instead of a haphazard art. It allows developers to build AI systems characterized by safety, reliability, and fairness. By providing meticulous assessment and comprehensive analysis of every dimension of AI models and their data, Kolena adopts a highly granular approach: unit testing for machine learning. It ensures that AI models undergo rigorous testing at the scenario level before their deployment to users, significantly reducing risk to the business. That kind of peace of mind will catalyze adoption by bigger businesses, regulated sectors, and industries like healthcare that have no margin for error.
When we led Kolena’s seed round, we spoke with many practitioners from the industry. It was clear that there was a need but no competing tooling around building reliable, enterprise-ready AI products. One leader we spoke with from a well-known Silicon Valley company mentioned, “We do this using aggregate metrics currently, which leaves huge blind spots in our model validation process.” This is why customers were eager to use Kolena before the product was even built. They were dedicating significant headcount to ad hoc testing and were in such dire need of tooling that they considered building proprietary dashboards. This proved to be too complex, with big questions around data segmentation, testing diversity, and applying perturbations. Most enterprises preferred to buy rather than build, and now they have Kolena.
![]() |
As an AI-native venture fund that builds and tests its own models for investment sourcing and portfolio recruiting, SignalFire saw the need for Kolena early on and led its $6M seed round. We’ve since used our Beacon AI data platform to assist Kolena with commercial, technical, and leadership recruiting searches, and data pulls of potential customer lists. Now we’re excited to back its $15M Series A led by our friend David Hornik at Lobby Capital.
Kolena is fixing the broken testing workflow that even leading AI organizations like OpenAI are wasting time and risking mistakes by doing manually. It is tedious, time-consuming, and hard to manage at scale without the proper tooling. By giving time, energy, headcount, and assurance back to enterprises, Kolena is unlocking the next stage of AI adoption.
*Portfolio company founders listed above have not received any compensation for this feedback and may or may not have invested in a SignalFire fund. These founders may or may not serve as Affiliate Advisors, Retained Advisors, or consultants to provide their expertise on a formal or ad hoc basis. They are not employed by SignalFire and do not provide investment advisory services to clients on behalf of SignalFire. Please refer to our disclosures page for additional disclosures.
Related posts

Why we’re continuing to invest in Tofu’s vision - Reducing martech bloat for GTM teams
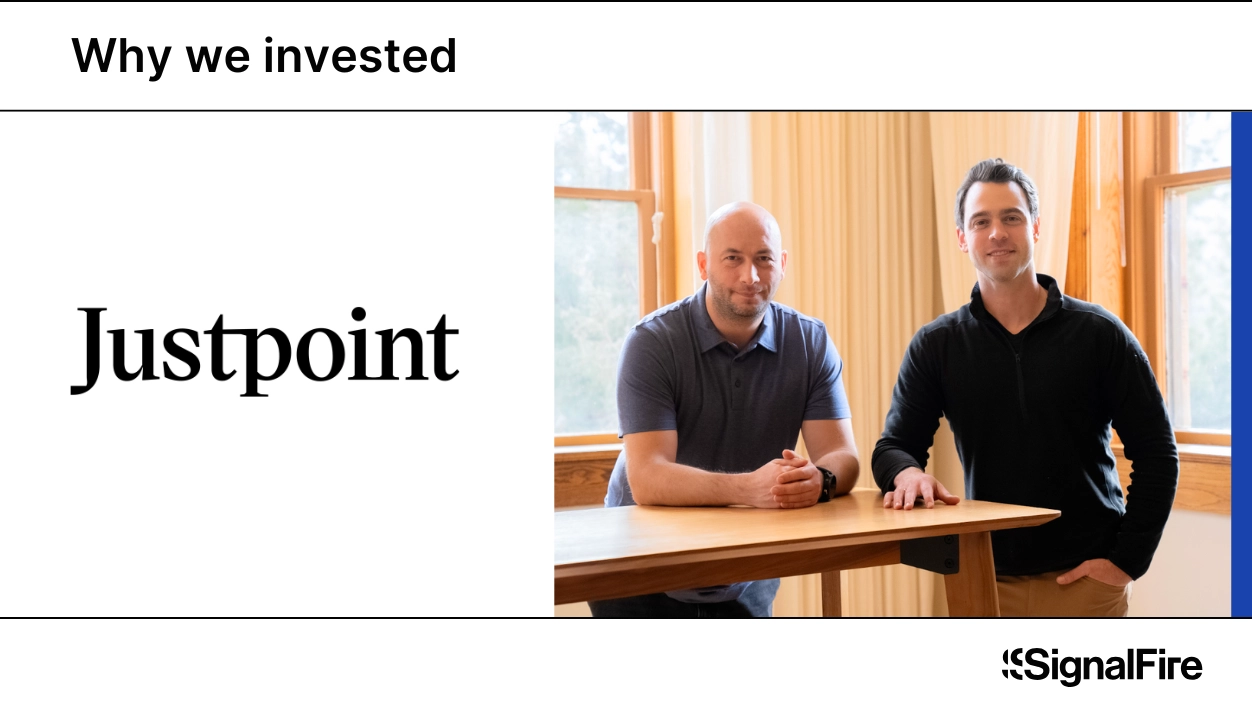
Justpoint raises $95M to tackle the toxic exposure epidemic with AI
